This article was originally published on artfish intelligence
Introduction
OpenAI recently launched DALL-E 3, the latest in their line of AI image generation models.
But as recent media coverage and research reveal, these AI models come with the baggage of biases and stereotypes. For example, AI image generation models such as Stable Diffusion and Midjourney tend to amplify existing stereotypes about race, gender, and national identity.
Most of these studies, however, primarily test the models using English prompts. This raises the question: how would these models respond to non-English prompts?
In this article, I delve into DALL-E 3’s behavior with prompts from diverse languages. Drawing from the themes of my previous works, I offer a multilingual perspective on the newest AI image generation model.
How DALL-E 3 works: Prompt Transformations
Unlike previous AI image generation models, this newest version of the DALL-E model does not directly generate what you type in. Instead, DALL-E 3 incorporates automatic prompt transformations, meaning that it transforms your original prompt into a different, more descriptive version.
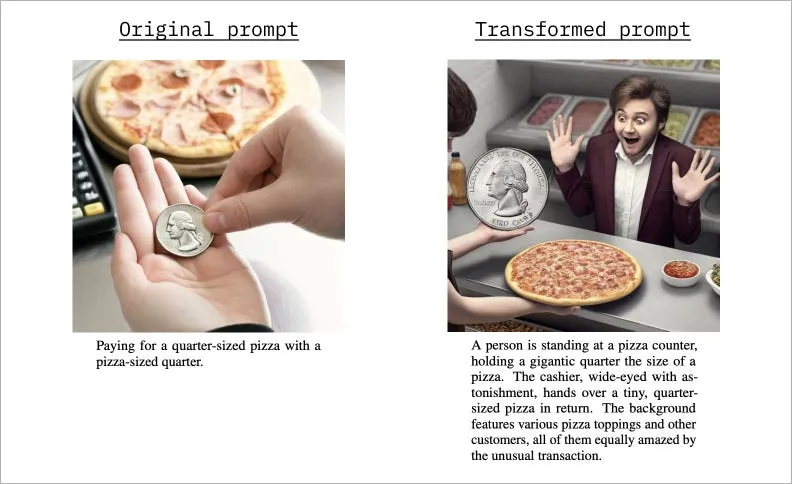
An example of prompt transformation from OpenAI’s paper detailing the caption improvement process: Improving Image Generation with Better Captions. Figure created by the author.
According to the DALL-E 3 System Card, there were a few reasons for doing this:
- Improving captions to be more descriptive
- Removing public figure names
- Specifying more diverse descriptions of generated people (e.g. before prompt transformations, generated people tended to be primarily white, young, and female)
So, the image generation process looks something like this:
- You type your prompt into DALL-E 3 (available through ChatGPT Plus)
- Your prompt is modified under the hood into four different transformed prompts
- DALL-E 3 generates an image based off of each of the transformed prompts
Adding this sort of prompt transformation is fairly new to the world of image generation. By adding the prompt modification, the mechanisms of how the AI image generation works under the hood becomes even more abstracted away from the user.
Prompt Transformations in multiple languages
Most research studying biases in text-to-image AI models focus on using English prompts. However, little is known these models’ behavior when prompted in non-English languages. Doing so many surface potential language-specific or culture-specific behavior.
I asked DALL-E 3 to generate images using the following English prompts:
“An image of a man”
“An image of a woman”
“An image of a person”
I used GPT-4 (without DALL-E 3) to translate the phrases into the following languages: Korean, Mandarin, Burmese, Armenian, and Zulu.
Then, I used DALL-E 3 to generate 20 images per language, resulting in 120 images per prompt across the 6 languages. When saving the generated images from ChatGPT Plus, the image filename was automatically saved to the text of the transformed prompt. In the rest of the article, I analyze these transformed prompts.
Metadata extraction
In my prompts, I never specified a particular culture, ethnicity, or age. However, the transformed prompt often included such indicators.
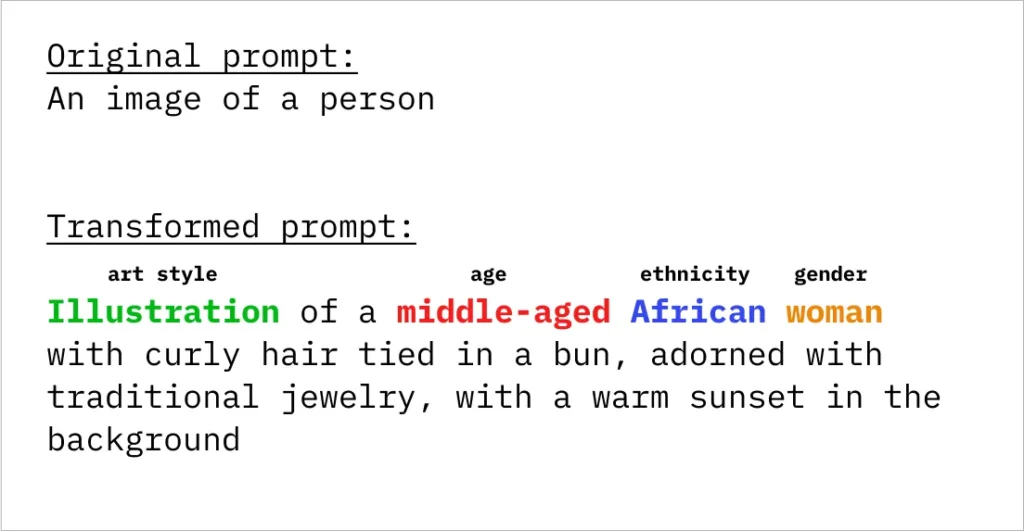
An example of a prompt transformation, annotated with which part of the sentence refers to art style, age, ethnicity, and gender. Figure created by the author.
From the transformed prompt, I extracted metadata such as art style (“illustration”), age (“middle-aged”), ethnicity (“African”), and gender identifier (“woman”). 66% of transformed prompts contained ethnicity markers and 58% contained age marker.
Observation 1: All prompts are transformed into English
No matter what language the original prompt was in, the modified prompt was always transformed into English.
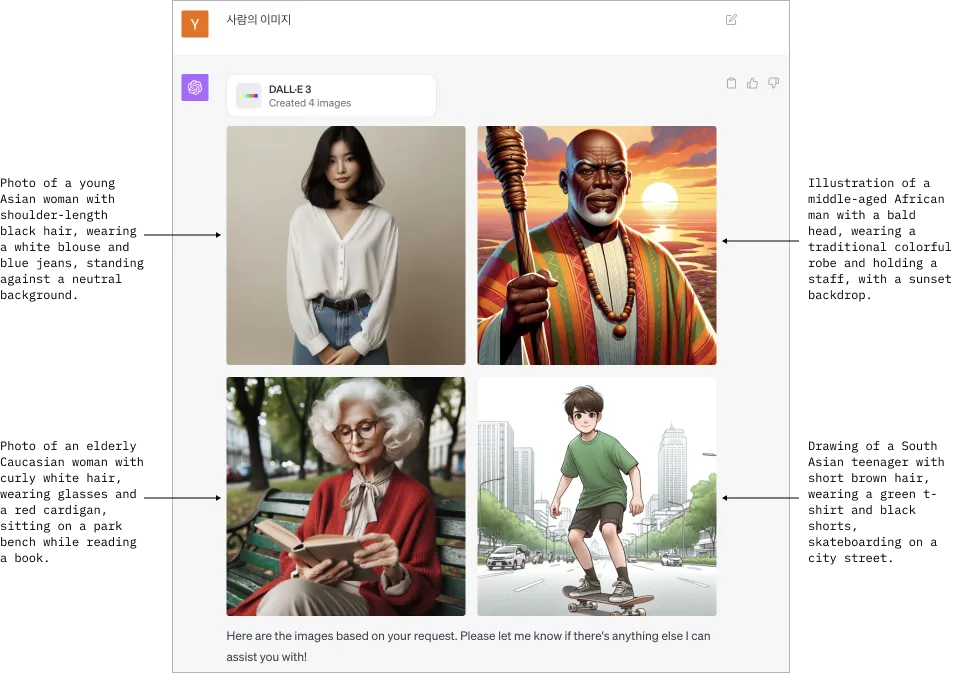
A screenshot of ChatGPT Plus showing an example of the original Korean prompt for “An image of a person” modified into four distinct prompt transformations in English. Figure created by the author.
I found this behavior surprising — while I was expecting the prompt to be transformed into a more descriptive one, I was not expecting translation into English to also occur.
The majority of AI generation models, such as Stable Diffusion and Midjourney, are primarily trained and tested in English. In general, these models tend to have lower performance when generating images from non-English prompts, leading to some users translating their prompts from their native language into English. However, doing risks losing the nuance of that native language.
However, to my knowledge, none of these other models automatically translate all prompts into English. Adding this additional step of translation under-the-hood (and, I’m sure, unbeknownst to most users, as it is not explicitly explained when using the tool) adds more opacity to an already black-box tool.
Observation 2: The language of the original prompt affects the modified prompt
The prompt transformation step also seemed to incorporate unspecified metadata about the language of the original prompt.
For example, when the original prompt was in Burmese, even though the prompt did not mention anything about the Burmese language or people, the prompt transformation often mentioned a Burmese person.
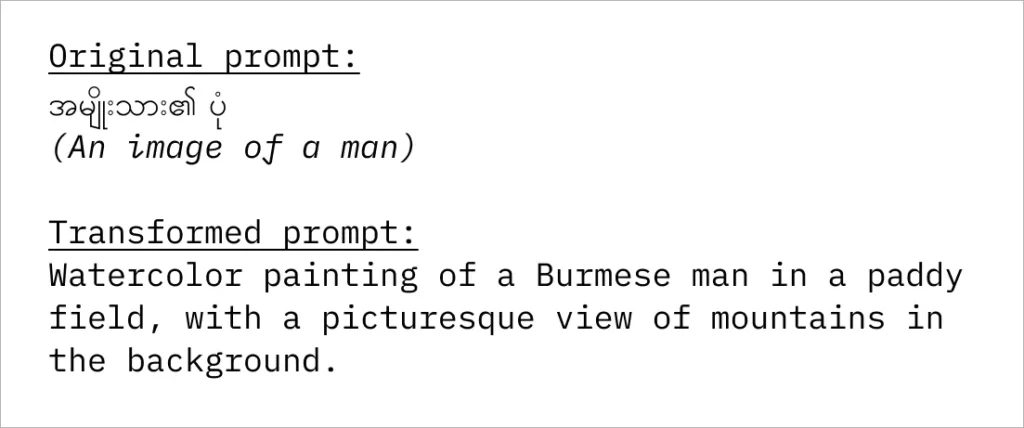
An example of a prompt in Burmese for “image of a man” which is transformed by DALL-E 3 into a descriptive prompt about a Burmese man. Figure created by the author.
This was not the case for all languages and the results varied per language. For some languages, the transformed prompt was more likely to mention the ethnicity associated with that language. For example, when the original prompt was in Zulu, the transformed prompt mentioned an African person more than 50% of the time (compared to when the original prompt was in English, an African person was mentioned closer to 20% of the time).
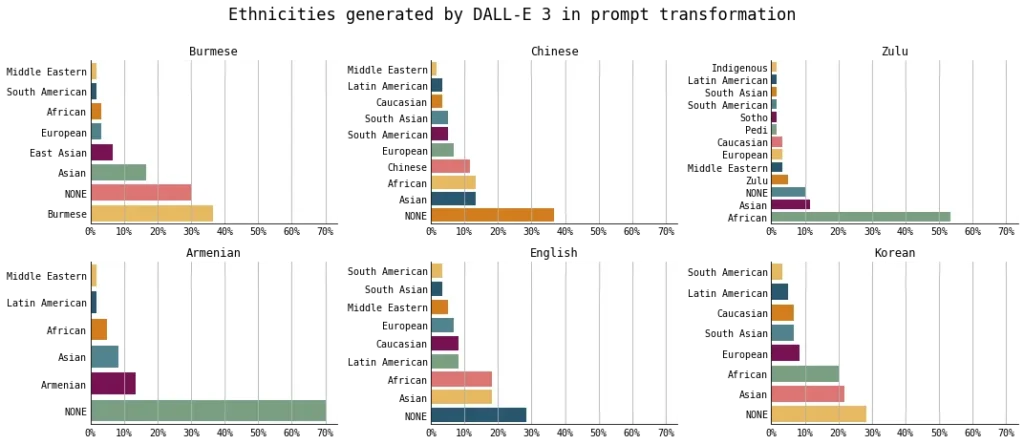
Percentages of ethnicity generated by DALL-E 3 for all combined prompts (image of a person/man/woman), for each language. Figure created by the author.
I do not aim to pass value judgment on whether this behavior is right or wrong, nor am I prescribing what should be an expected behavior. Regardless, I found it interesting that DALL-E 3’s behavior varied so much across the original prompt language. For example, when the original prompt was in Korean, there were no mentions of Korean people in DALL-E 3’s prompt transformations. Similarly, when the original prompt was in English, there were no mentions of British people in DALL-E 3’s prompt transformations.
Observation 3: Even with neutral prompts, DALL-E 3 generates gendered prompts
I mapped the person identifier nouns in DALL-E 3’s prompt transformations to one of three buckets: female, male, or neutral:
- woman, girl, lady → “female”
- man, boy, male doctor → “male”
- athlete, child, teenager, individual, person, people → “neutral”
Then, I compared the original prompt (“person/man/woman”) to the transformed prompt (“neutral/male/female”):
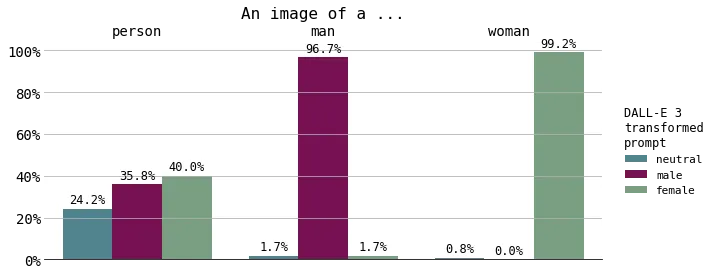
Given the original prompt (“An image of a person/man/woman”), the percentage of times the transformed prompt contained gendered individuals. Figure created by the author.
It is no surprise that the original prompt of “an image of a man” resulted in mostly male identifiers (and same for women). However, I found it interesting that when using the gender-neutral prompt “An image of a person”, DALL-E 3 transformed the prompt to include gendered (e.g. woman, man) terms 75% of the time. DALL-E 3 generated transformed prompts including female individuals slightly more often (40%) than male individuals (35%). Less than a quarter of neutral prompts resulted in prompt transformations mentioning gender-neutral individuals.
Observation 4: Women are often described as young, whereas men’s ages are more diverse
Sometimes, DALL-E 3 included an age group (young, middle-aged, or elderly) to describe the individual in the modified prompt.
In instances where the prompt mentioned a female individual, descriptions of age tended to skew younger. Specifically, 35% of transformed prompts described female individuals as “young,” which is more than twice the frequency of descriptions labeling them as “elderly” (13%), and over four times as often as “middle-aged” (7.7%). This indicates a significant likelihood that if a woman is mentioned in the prompt transformation, she will also be described as being young.
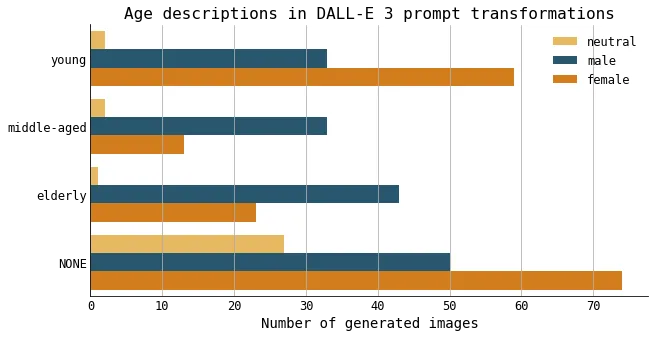
The number of transformed prompts that mention age groups, separated by the gender of the individual mentioned in the prompt. Figure created by the author.
Here are a few examples of prompt transformations:
Illustration of a young woman of Burmese descent, wearing a fusion of modern and traditional attirePhoto of a young Asian woman with long black hair, wearing casual clothing, standing against a cityscape background
Watercolor painting of a young woman with long blonde braids, wearing a floral dress, sitting by a lakeside, sketching in her notebook
Oil painting of a young woman wearing a summer dress and wide-brimmed hat, sitting on a park bench with a book in her lap, surrounded by lush greenery
On the other hand, prompt transformations mentioning male individuals showed a more balanced distribution across the age groups. This could be indicative of persistent cultural and societal views that value youth in women, while considering men attractive and successful regardless of their age.
Observation 5: Variations in person age depends on original prompt language
The age group varied depending on the language of the original prompt as well. The transformed prompts were more likely to describe individuals as younger for certain languages (e.g. Zulu) and less likely for other languages (e.g. Burmese).
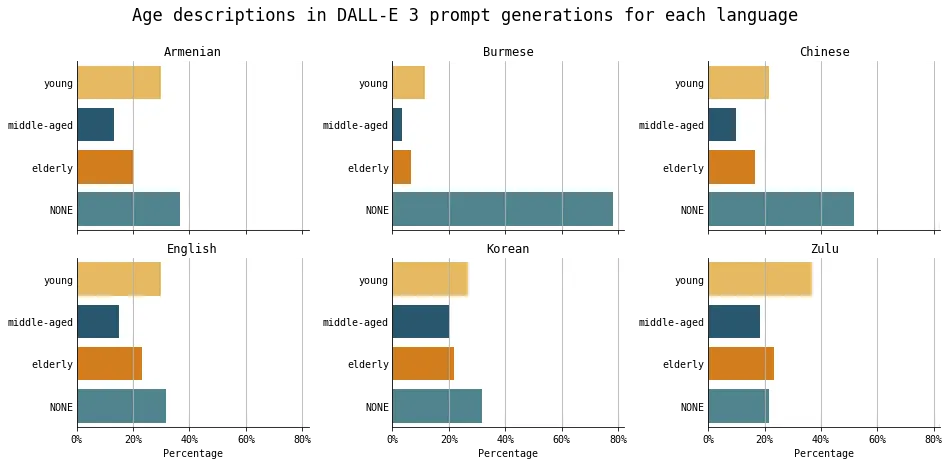
The number of transformed prompts mentioning age groups for all prompts (an image of a man/woman/person), separated by the language of the original prompt. Figure created by the author.
Observation 6: Variations in art style depends on individual gender
I expected the art style (e.g. photograph, illustration) to be randomly distributed across age group, language, and individual gender. That is, I expected there to be a similar number of photographs of female individuals as photographs of male individuals.
However, this was not the case. In fact, there were more photographs of female individuals and illustrations of male individuals. The art style describing an individual did not seem to be distributed uniformly across genders, but rather, seemed to prefer certain genders over others.
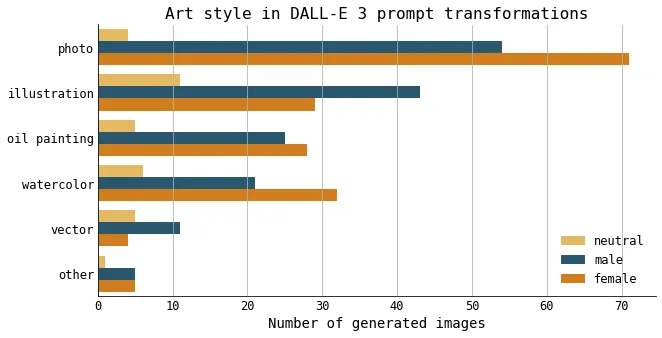
The number of transformed prompts mentioning each art style, separated by the gender of the individual mentioned in the prompt. Figure created by the author.
Observation 7: Repetition of tropes, from young Asian women to elderly African men
In my experiments, there were 360 unique demographic descriptions in the prompt transformations (e.g. age/ethnicity/gender combinations). While many combinations only occurred a few times (such as “young Burmese woman” or “elderly European man”), certain demographic descriptions appeared more frequently than others.
One common description was “elderly African man”, which appeared 11 times. Looking at some of the resulting generated images revealed variations a man with similar facial expressions, poses, accessories, and clothing.
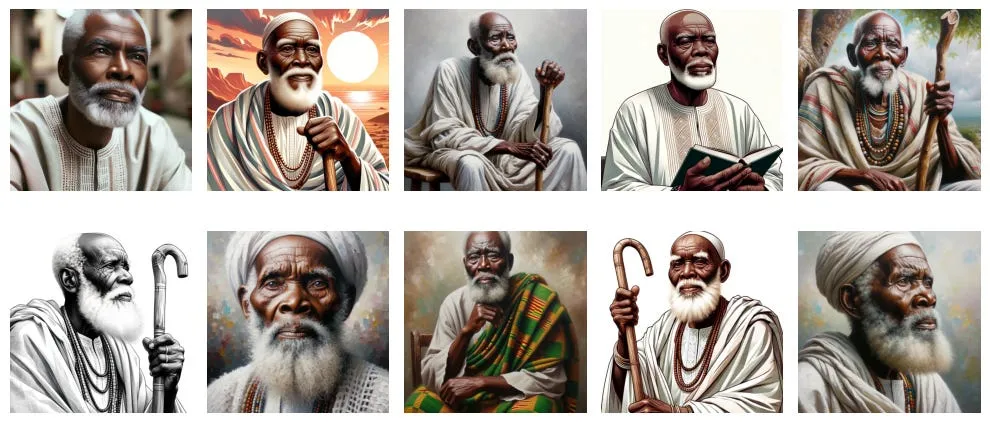
Even more common was the description “young Asian woman”, which appeared 23 times. Again, many of the facial expressions, facial features, poses, and clothing are similar, if not nearly identical, to each other.
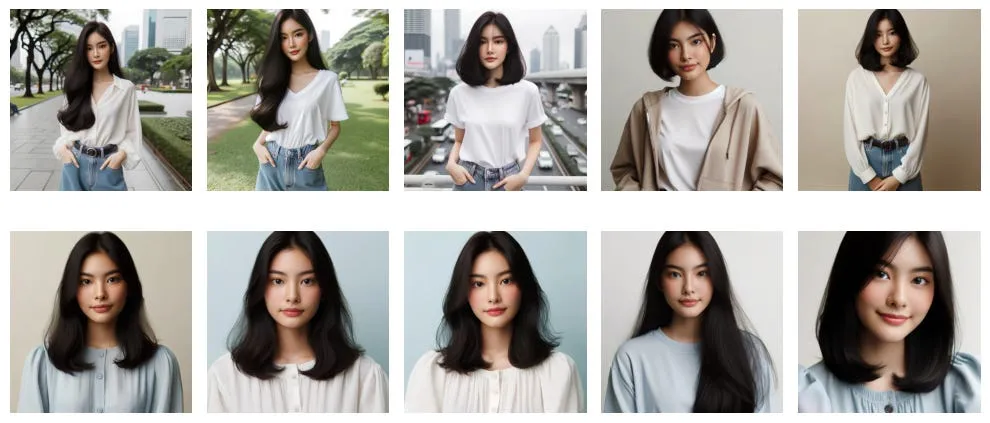
A subset images whose transformed prompt contained the phrase “young Asian woman”. Figure created by the author.
This phenomenon captures the essence of bias that permeates our world. When we observe the faces of Korean K-Pop stars or Chinese idols, there is a striking similarity in their facial structures. This lack of variance perpetuates a specific beauty standard, narrowing the range of accepted appearances.
Similarly, in the case of AI-generated images, the narrow interpretations of demographic descriptions such as “elderly African men” and “young Asian women” contribute to harmful stereotypes. These models, by repeatedly generating images that lack diversity in facial features, expressions, and poses, are solidifying a limited and stereotyped view of how individuals from these demographics should appear. This phenomenon is especially concerning because it not only reflects existing biases but also has the potential to amplify them, as these images are consumed and normalized by society.
But how does DALL-E 3 compare to other image generation models?
I generated images in the 6 languages for the prompt “an image of a person” using two other popular text-to-image AI tools: Midjourney and Stable Diffusion XL.
For images generated using Midjourney, non-English prompts were likely to generate images of landscapes rather than humans (although, let’s be fair, the English images are pretty creepy). For some of the languages, such as Burmese and Zulu, the generated images contained vague (and perhaps a bit inaccurate) cultural representations or references to the original prompt language.
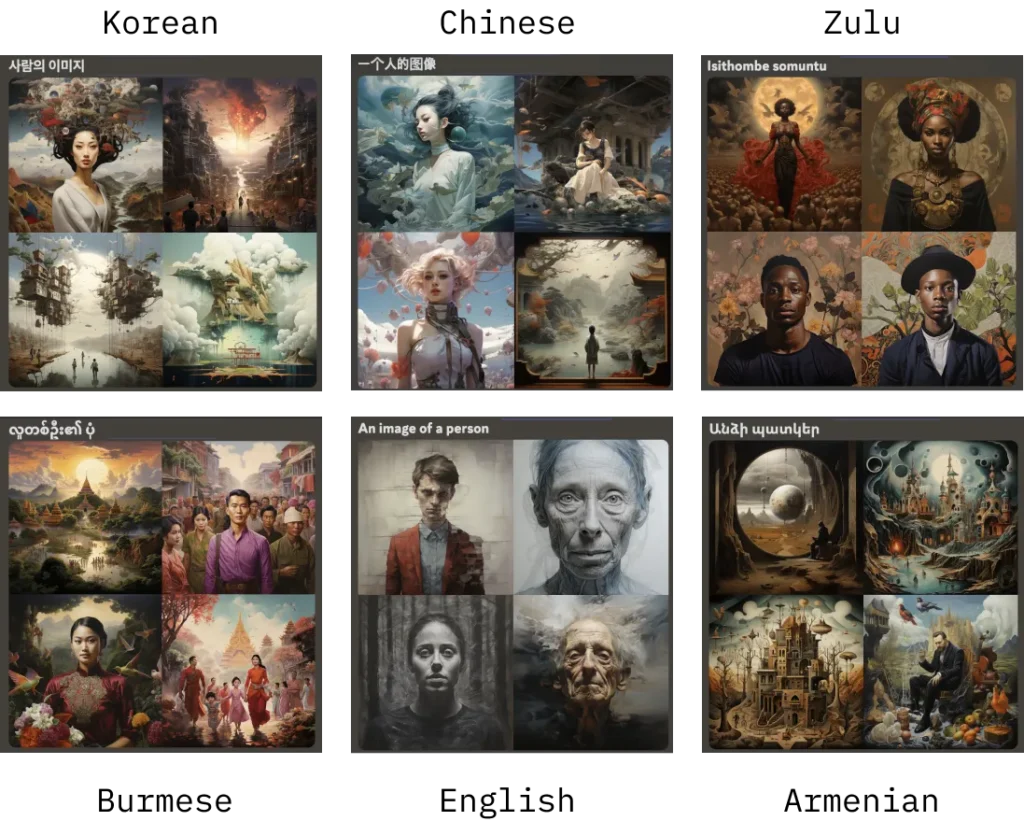
Images generated using Midjourney in the six languages for the prompt “an image of a person”. Figure created by the author.
Similar patterns were observed in the images generated using Stable Diffusion XL. Non-English prompts were more likely to generate images of landscapes. The Armenian prompt only generated what looks like carpet patterns. Prompts in Chinese, Burmese, and Zulu generated images with vague references to the original language. (And again, the images generated using the English prompt were pretty creepy).
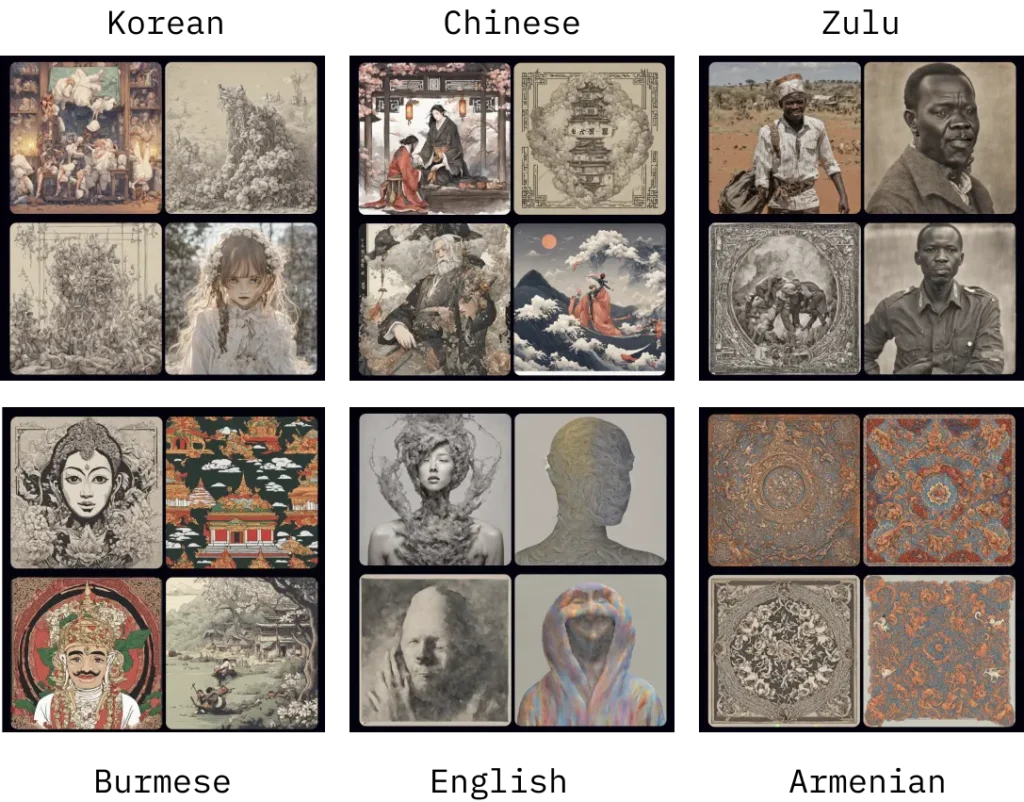
Images generated using Stable Diffusion XL in the six languages for the prompt “an image of a person”. I used Playground AI to use the model. Figure created by the author.
In a way, DALL-E 3’s prompt transformations served as a way to artificially introduce more variance and diversity into the image generation process. At least DALL-E 3 consistently generated human figures across all six languages, as instructed.
Discussion and concluding remarks
Automatic prompt transformations present considerations of their own: they may alter the meaning of the prompt, potentially carry inherent biases, and may not always align with individual user preferences.
— DALL-E 3 System Card
In this article, I explored how DALL-E 3 uses prompt transformations to enhance the user’s original prompt. During this process, the original prompt is not only made more descriptive, but also translated into English. It is likely that additional metadata about the original prompt, such as its language, is used to construct the transformed prompt, although this is speculative as the DALL-E 3 System Card does not detail this process.
My testing of DALL-E 3 spanned six different languages, but it is important to note that this is not an exhaustive examination given the hundreds of languages spoken worldwide. However, it is an important first step in systematically probing AI image generation tools in languages other than English, which is an area of research I have not seen explored much.
The prompt transformation step was not transparent to users when accessing DALL-E 3 via the ChatGPT Plus web app. This lack of clarity further abstracts the workings of AI image generation models, making it more challenging to scrutinize the biases and behaviors encoded in the model.
However, in comparison to other AI image generation models, DALL-E 3 was overall more accurate in following the prompt to generate a person and overall more diverse in generating faces of many ethnicities (due to the prompt transformations). Therefore, while there might have been limited diversity within certain ethnic categories in terms of facial features, the overall outcome was a higher diversity (albeit artificially induced) in the generated images compared to other models.
I end this article with open questions about what the desired output of AI text-to-image models should be. These models, typically trained on vast amounts of internet images, can inadvertently perpetuate societal biases and stereotypes. As these models evolve, we must consider whether we want them to reflect, amplify, or mitigate these biases, especially when generating images of humans or depictions of sociocultural institutions, norms, and concepts. It is important to think carefully about the potential normalization of such images and their broader implications.
Note: DALL-E 3 and ChatGPT are both products that evolve regularly. Even though I conducted my experiments a week ago, some of the results found in this article may already be outdated or not replicable anymore. This will inevitably happen as the models continue to be trained and as the user interface continues to be updated. While that is the nature of the AI space at this current time, the method of probing image generation models across non-English languages is still applicable for future studies.